Editor’s note: From equipment failures to cyber attacks, unexpected events can cripple businesses. But what if you could anticipate and prevent the failure instead of reacting to it? Enters AI-driven anomaly detection in manufacturing industry for proactively mitigating risks.
In the upcoming blog, you are going to discover how AI-driven anomaly detection can empower your company to become more resilient, efficient, and future-ready to mitigate risks costing the manufacturers millions of dollars effectively.
Why traditional risk mitigation in manufacturing operations isn’t enough?
Whether it is inconsistencies in production line, variations in input material quality or properties, human errors, procedural lapse, or even data breaches, these risks can impact the manufacturers with reduced productivity, increased costs, missed delivery targets, regulatory non-compliance, and harm to brand reputation.
Acknowledging the cost of risk in the manufacturing industry, 58% of organizations have set up a plan to respond to risks. Hence, the industry is adopting a proactive approach to risk mitigation by utilizing AI/ML-powered anomaly detection as a counteractive solution.
Even though the traditional risk mitigation methods also analyze the incoming data and compare them to the established baseline of normal behavior, they often rely on pre-defined thresholds for identifying risks and wait for the event to occur that crosses a specific limit before triggering the alert.
Here, anomaly detection overcomes the reactiveness of traditional methods through:
Anomaly detection has emerged as a powerful risk-mitigation tool for identifying risks before they cause significant issues. By recognizing deviations from normal patterns in operations, the data from the process empowers the manufacturers to address risks and problems pre-emptively and optimize processes facility-wide.
Application of anomaly detection in manufacturing industry for proactive risk mitigation
Manufacturers are now saving billions of dollars by gaining more advanced warnings to substantially expand risk mitigation by leveraging the potential of AI/ML to train their anomaly detection models. They can now perform an array of complex tasks depending on the case.
Let us talk about the fundamental ones here.

1. Using predictive maintenance to improve asset availability
A study says predictive maintenance can save manufacturers 1.6 million hours of downtime annually and $734 billion through a 6% increase in productivity.
Predictive Maintenance generally, deals with anomalies from the machine (vibration analysis, temperature monitoring, sensor data analysis), process (deviations from control parameters, energy consumption spikes), and other sources (Human errors, data threats).
Hence to minimize financial implications, balance extended equipment life with machine downtime risk, and improve uptime through periodic part replacement, a comprehensive risk mitigation strategy combining data-driven anomaly detection for predictive maintenance will empower manufacturers to improve the remaining useful life of their machines and at the same time avoid unplanned downtime. This resulted in reduced downtime by 30 to 50 percent and increased machine life by 20 to 40 percent.
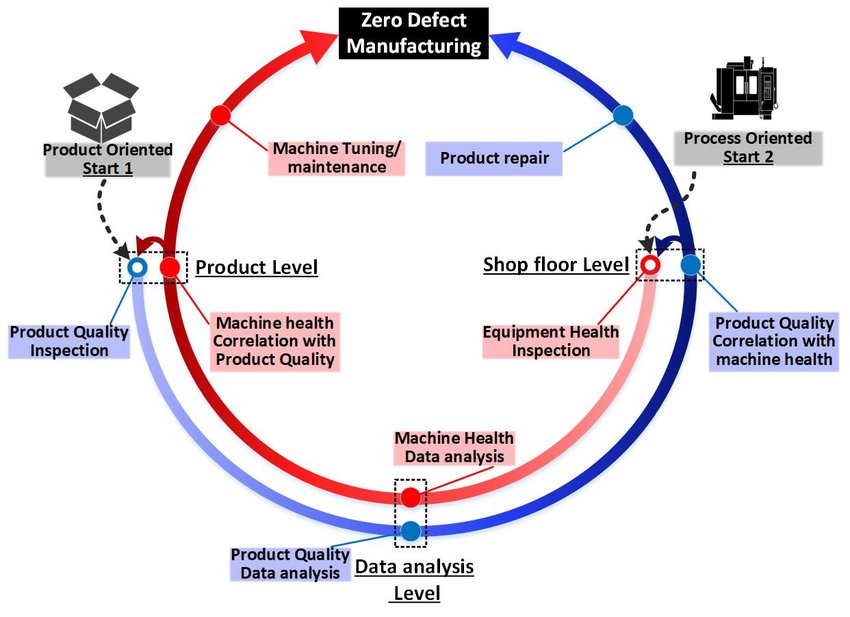
Here, anomaly detection to determine whether the product quality fulfils the required requirements become a vital parameter for analysing equipment health. If the product quality fulfils the requirements, the related machine's state is considered healthy; if not, anomaly detection identifies and warns that the corresponding machine requires maintenance and/or (re)calibration or tuning based on defect data. In contrast, for the process-oriented approach, the machine's health state is monitored; if it is good, the finished output will be of high quality and vice versa.
The implementation is done as a closed-loop process with feedback loops on either the product or the process quality, resulting in maintenance, machine (re)calibration, and/or tuning in the event of low quality. Doing so help evade risks by early detection of warning indications, such as unexpected vibrations, temperature variations, or pressure levels, allowing maintenance teams to avert any disruptions. This allows for prompt intervention and preventive maintenance by routine scheduling, reducing the chances of any discrepancy.
2. Fraud Detection and activating countermeasures
Due to unmonitored supply networks, underlying assets in the form of inventory, and numerous, regular transactions that put it at risk of fraud, the manufacturing sector is frequently one of the most vulnerable. Manufacturing companies have recently been intimidated by some of the frauds listed below.

The fact is that a huge toll is being caused by these frauds.
According to the Association of Certified Fraud Examiners, each fraud event costs $200,000 on average and the median loss for the manufacturing industry is an astounding $177,000.
While it is impossible to eliminate these frauds entirely, the industry has now realized the potential of advanced anomaly detection and has put data to work against fraud. By using unsupervised learning techniques, it makes detection more effective, especially utilizing large data sets.
Forward-thinking firms hence use advanced anomaly detection techniques and tools instead of manually analyzing dashboards and reports.
Advanced anomaly detection algorithms can identify trends and correlations, making it easier to determine whether abnormalities are real. This data-driven approach constantly analyses transaction data for any suspicious deviations by:
In short, manufacturers can quickly discover such problems and reduce risks early on.
By continuously learning from each occurrence, the system streamlines the detection procedure by improving its prediction abilities. As a result, over time, this strengthens defences against new threats by reducing financial losses and giving time to evaluate and deploy proper risk mitigation measures.
Protection against Cyber security threats–
The manufacturing industry had the largest percentage of cyberattacks among the world's primary industries. Manufacturing organizations were subjected to roughly a quarter, or 25%, of all cyberattacks during 2022.

Therefore, for proper risk mitigation, it becomes vital for manufacturers to understand what makes the industry such an appealing target for cyber criminals.
Reasons for Targeted Cyber crime in the Manufacturing Industry
Since data breaches pose a significant risk, anomaly detection models and tools supporting zero trust security model has become popular as part of their risk mitigation strategy.
How it works
Anomaly detection models and tools in the zero-trust architecture evaluate risk and determine risk score each time a user requests access to an application. The algorithm allows systems to rapidly consider multiple data points and determine whether to allow or deny access. When no anomaly is detected, the system can automatically provide access; when an anomaly is detected, it triggers an alert to the system administrator.
Since cyber-attacks can be based on the requests for access from users(Attacks have a higher frequency), Network anomaly detection models use anomalous data from intrusion detection systems to identify the underlying patterns and trends and combine it with a Zero Trust model to alert administrators when an intruder attempts to breach the security perimeter by tracking the traffic and monitoring the safety of an organization’s network security,
How anomaly detection helps in risk mitigation
When advanced anomaly detection is combined with zero trust architecture, it improves real-time risk detection across a request's lifespan, allowing organizations to:
3. Quality Control and Management
Manufacturing companies are increasingly utilizing alternative quality management methods, such as Zero-Defect Manufacturing (ZDM), to enhance their competitiveness, productivity, profitability, and sustainability.
Why is zero defect manufacturing with anomaly detection a viable solution?
It is important to clarify that Zero defect manufacturing isn't necessarily a "replacement" for traditional quality improvement approaches like Six Sigma, Lean, or Total Quality Management (TQM). Instead, it can be seen as a complementary philosophy or goal that leverages these established methodologies to achieve near-perfect quality.
Traditional approach | Zero defect manufacturing | Anomaly detection process in Zero defect manufacturing |
---|---|---|
Six sigma | ZDM shares Six Sigma's focus on data-driven process improvement but emphasizes defect prevention over defect reduction. Six Sigma's DMAIC methodology can be valuable for identifying and eliminating the root causes of defects. | While six sigma is still valuable for root cause analysis, anomaly detection in ZDM offers a real-time and proactive edge for defect prevention. |
Lean | ZDM aligns with Lean's commitment to eliminating waste and optimizing processes. However, Lean might prioritize flow efficiency overachieving absolute zero defects. | Anomaly detection complements Lean's waste reduction by focusing on preventing defects at the source, enhancing overall efficiency. |
Theory of Constraints (TOC) | ZDM shares TOC's focus on identifying and resolving bottlenecks to improve overall performance. However, TOC focuses on optimizing specific constraints, while ZDM aims for defect-free production across the entire process. | Anomaly detection can identify and address bottlenecks by real-time monitoring of deviations, aiding in optimizing the entire production process for zero defects. |
Total Quality Management (TQM) | Both ZDM and TQM strive for continuous improvement and customer satisfaction. However, TQM might accept a certain level of defects as inevitable, while ZDM aims for complete elimination. | Both methodologies share continuous improvement goals, but anomaly detection offers a data-driven and targeted approach for defect elimination, aligning with TQM's emphasis on data-based decision-making. |
How it works
Zero Defect Manufacturing uses a two-pair process (Detect-Predict and Repair-Prevent). By leveraging advanced anomaly detection in this two-pair process, it proactively identifies and adapts to the flaws found in the system which may cause defects to happen. Sensors embedded in equipment further continuously collect data on vibrations, temperature, and power consumption. Anomaly detection algorithms analyze this data, flagging potential equipment failures before they occur, and preventing costly downtime and production disruptions.
How it helps in risk mitigation
Early anomaly detection paired with responsive problem-solving of zero-defect manufacturing will eliminate the risk of rework, and delays in shipping and recalls by fine-tuning the quality control process and notably improve customer satisfaction thanks to high product quality
4. Anomaly detection for better supply chain visibility
Today manufacturing supply chain generates a staggering 1,812 petabytes (PB) of data every year. While valuable insights lie within this data, manually analyzing it is practically impossible. Due to these anomalies manufacturers face rising risks including longer decision cycles, lack of real-time visibility, and vulnerability to disruptions. However, AI-powered anomaly detection can help uncover critical signals faster within vast data streams.
1. Point-of-sale (POS): Due to the bullwhip effect, a distortion of actual customers’ demand is noticed. Anomaly detection here can help by analyzing sales data from various locations or distribution centers. By monitoring sales patterns and comparing them to historical data, anomalies such as sudden spikes or drops in sales can be identified. These anomalies may indicate issues such as stockouts, theft, or fraudulent activities. By detecting these anomalies, manufacturers can take corrective actions, such as replenishing inventory or investigating potential fraud, to ensure smooth operations and minimize financial losses.
2. Inventory planning and management: Anomaly detection can help optimize inventory levels by flagging unusual fluctuations in stock levels or unexpected patterns in demand. By identifying anomalies in inventory data, manufacturers can make informed decisions to prevent stockouts, reduce excess inventory, and enhance supply chain efficiency.
Check out how inventory management firms are winning with data analytics and automation.
3. Transportation planning and management: When it comes to transportation, companies make sure to maximize the filling capacities of the vehicles and to limit unnecessary kilometres. The anomalies here not only hinder timely deliveries but also pose risks to customer satisfaction and overall supply chain resilience. Anomaly detection, powered by advanced artificial intelligence algorithms, emerges as a proactive solution to address these challenges. By analyzing vast datasets from various sources such as GPS tracking, weather forecasts, and real-time traffic updates, anomaly detection algorithms can swiftly identify irregular patterns or deviations from the norm. This enables transportation planners to anticipate and mitigate potential disruptions, optimizing routes and schedules in real-time to ensure a smoother, more efficient transportation process and enhancing the overall reliability of the supply chain.
4. Vendor Risk Evaluation: The complexity of evaluating vendor performance and identifying potential risks becomes difficult to monitor as large volume of data points related to vendor activities, performance metrics, and external factors that may impact vendor reliability. In such cases anomaly detection algorithms can quickly identify unexpected changes and irregularities in vendor behaviour or performance metrices. This allows organizations to create customized risk profiles for each vendor based on historical data and specific performance metrics. This tailored approach enhances the accuracy of risk assessment and enables a more nuanced understanding of vendor behavior.
Wrapping up
The types of risks that a manufacturer may face are changing, but with improved anomaly detection, so will you. At Polestar solutions, we specialize in developing solutions that include advanced analytics and AI, hence improving the resilience and intelligence of your business tools.
Our services enhance your business tools with advanced predictive analytics, real-time risk monitoring, and adaptive decision-making capabilities, ensuring a proactive approach to risk management and fostering operational agility to address today's issues while preparing for a future in which anomaly detection will be synonymous with anticipatory excellence in the manufacturing sector.