Editor’s Note: Do you want your manufacturing defects to not just minimized, but eradicated?
In this blog discover how intelligent algorithms can predict, optimize and transform processes in real-time with AI in zero defect manufacturing. Dive in and unlock the path to a production line so flawless, it'll leave you with only one regret: not embracing AI sooner.
Manufacturing, on its road to becoming the world's largest market, developed an issue with global consequences: 20% of every dollar spent in the industry is wasted.
This necessitates breaking down data silos and achieving complete integration and interoperability of manufacturing systems, proving that traditional quality-oriented manufacturing practices do not meet the industry 4.0 vision.
Here, the concept of zero-defect embraces numerous ideas and concepts from traditional quality improvement methods and goes beyond incorporating technological advancements like machine learning, deep learning, and artificial intelligence.
Why should manufacturers implement AI-driven zero-defect manufacturing?
Although AI as a concept and as a technology has been around for many years in various commercial product iterations, recent breakthroughs in supporting infrastructure and AI-specific products have sparked a new wave of adoption in the manufacturing industry. The interconnection of more robust computer platforms, more powerful communication networks, and datasets large enough for AI engines to process data in meaningful ways has resulted in the creation of better conditions for AI adoption, evident by the new wave of overall adoption evidenced in the industry.

Utilizing AI judiciously is now allowing manufacturers to unlock substantial quality, efficiency, and reliability improvements. This breaks the notion of achieving near-zero defect manufacturing as a difficult procedure happening only for products that have undergone extensive research.
Therefore, realizing its full potential requires moving beyond siloed applications in the manufacturing process toward an integrated AI strategy aligned with business goals. With the right approach, manufacturers can leverage AI to attain the next level of operational excellence and move closer to making zero defects a reality.
Why Artificial Intelligence helps in improving zero defect manufacturing?
The industry understands the benefits of implementation of zero-defect manufacturing but also realizes that it is not an easy procedure. Hence, Manufacturers have put their trust in data and AI. They are seeing visible results with AI incorporation like a 30%-50% decrease in downtime and a 10%-20% decrease in quality-related expenses by addressing the zero-defect manufacturing challenges.
Let’s find out how the industry is leveraging AI adoption in zero-defect manufacturing for improved results.

1. Predictive Maintenance for Reducing Downtime
According to a report, unplanned machine downtime costs manufacturers $260,000 for every hour of lost production, and 82% of manufacturers experience machine downtime at least once each year.
Zero defect manufacturing aims to produce products without any defects or flaws. However, even in such a high-quality manufacturing environment, equipment and machinery may still face downtime issues due to various reasons. Predictive maintenance can play a crucial role in minimizing downtime and addressing potential issues before they lead to defects.
Issue | Solution with Predictive Maintenance |
---|---|
Equipment Failure Prediction | Predictive maintenance uses data analytics and sensor technologies to monitor equipment health. By analysing patterns and detecting early signs of potential failures, maintenance activities can be scheduled proactively, minimizing the risk of unexpected breakdowns. |
Component Wear and Tear | Predictive maintenance monitors the condition of components, predicting when they are likely to wear out. This enables scheduled replacements or maintenance, preventing unexpected failures and maintaining production quality. |
Lubrication Issues | Predictive maintenance systems can monitor lubrication levels and conditions. Alerts can be generated when lubrication is insufficient, prompting timely maintenance to ensure smooth equipment operation. |
Temperature and Vibration Monitoring | Predictive maintenance systems utilize sensors to monitor temperature and vibration patterns. Deviations from normal ranges trigger alerts, allowing maintenance teams to investigate and address issues before they escalate. |
Energy Efficiency and Performance | Predictive maintenance analyzes performance data to identify inefficiencies and deviations from optimal operating conditions. This enables proactive adjustments to maintain energy efficiency and prevent degradation in product quality. |
Predictive maintenance can save manufacturers 1.6 million hours of downtime annually and $734 billion through a 6% increase in productivity.
Thanks to AI-powered predictive maintenance (PdM) and its transformative application of IoTs, manufacturers are improving efficiency while reducing the cost of machine failure. AI-powered predictive maintenance analyses sensor data (sensors can be installed on machinery and track vital machine health indicators in real time) to forecast malfunctions using data analysis and machine learning algorithms.
Leveraging its potential Ford Motor Company optimized the performance of its manufacturing equipment. Ford's AI-powered solutions predict when maintenance is needed, ensuring that resources and spare parts necessary for repair are on hand resulting in reduced downtime and increasing efficiency.
PepsiCo’s Fayetteville, Frito-Lay plant also reported a 0.75% year-to-date equipment downtime and a 2.88% unplanned downtime. Addressing the issue, The Frito-Lay plant which produces upwards of 150 pounds of product per year, implemented its robust program of Predictive maintenance enabling them to have a real-time assessment of its equipment health to predict failures or its remaining lifespan.
2. Anomaly Detection for Improved Equipment Performance
AI-powered anomaly detection approaches have been shown to improve the speed, accuracy, and efficiency of monitoring huge quantities of data to respond proactively to potential outliers and issues. Their capacity to analyze and derive information from data in real time enables organizations to detect and identify anomalies effectively.
To achieve this, Zero Defect Manufacturing consists of four ZDM techniques, separated into two categories: triggers and actions. The flowchart below depicts it’s working.
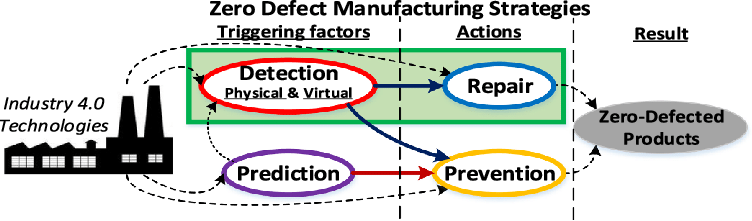
It then employs advanced anomaly detection to determine whether the product quality fulfills the required requirements. If the product quality fulfills it, the related machine's state is considered healthy; if not, anomaly detection identifies and warns that the corresponding machine requires maintenance and/or (re)calibration or tuning based on defect data. In contrast, for the process-oriented approach, the machine's health state is monitored; if it is good, the finished output will be of high quality and vice versa.
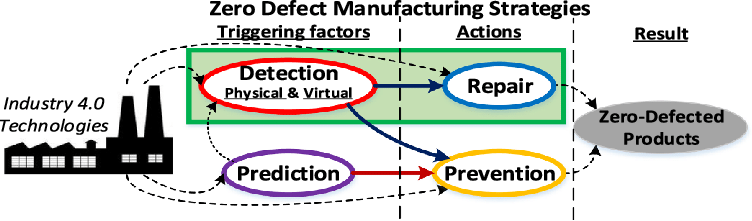
In both scenarios, ZDM implementation is a closed-loop process with feedback loops on either the product or the process quality, resulting in maintenance, machine (re)calibration, and/or tuning in the event of low quality.
AI-powered anomaly detection approaches have been shown to improve the speed, accuracy, and efficiency of monitoring huge quantities of data to respond proactively to potential outliers and issues. Their capacity to analyze and derive information from data in real time enables organizations to detect and identify anomalies effectively.
One of the most serious difficulties facing the manufacturing industry was that the traditional processes could only detect 20% of anomalies ahead of time.
The reason is straightforward – yesterday’s implementation of conditional monitoring architecture is ill-equipped to manage the torrent of data in today’s world. Therefore, addressing the issue, zero defect manufacturing as an approach - embraces the ideas and concepts from the traditional quality methods and uses anomaly detection methods to detect deviations from standard parameters to achieve the set requirements. This helps break data silos and achieve full integration and interoperability of manufacturing systems for effective detection.
How it works
By leveraging AI-powered anomaly detection in zero defect manufacturing’s pair process (Detect-Predict, Repair-Prevent, Predict-Prevent), it proactively identifies and adapts to the flaws found in the system that may cause defects to happen.
3. Automated Quality Checks with AI/ML
Many organizations in the industry will have true quality-related costs as high as 40% of total operations where the general thumb rule states that the cost of poor quality should be 10-15% of operations for a company to thrive. This proves that today, manufacturers have urgent needs to boost efficiency, decrease wastage, avoid recalls – and on top of that, make the end-to-end process better than before.
In response, zero defect manufacturing with AI systems powered by machine and visual visions, along with deep learning algorithms, has emerged as a transformative solution. These AI systems autonomously scan thousands of parts per minute with unparalleled precision, achieving near-perfect defect detection.
BMW iFactory using AIQX (Artificial Intelligence Quality Next) helps us get a better perspective. It uses AIQX to monitor the quality of products during production process, identifying and addressing quality issues before they become a major bottleneck. This technology not only addresses the limitations of manual inspection by leveraging AI/ML but also brings substantial improvements in defect detection, throughput, and overall operational efficiency.
Though adoption rates of automated visual inspection in manufacturing are currently low, at less than 10%; Automated visual inspection can reduce defect rates by up to 90%, with over 50% improvement in throughput.
This paradigm shift is crucial in meeting the evolving requirements of the industry, providing speed, and addressing unique circumstances that challenge traditional inspection methods. Historically, quality control (QC) relied on manual methods, but visual inspection has shown to be a game changer. Therefore, now by leveraging AI’s potential, manufacturers can not only mitigate risks associated with machine failures but also achieve long-term cost savings, improved equipment lifespan, and an improved environmental impact.
4. AI-driven Digital Twin for Optimizing Asset Performance
The integration of digital twins aligns with the industry 4.0 paradigm and Quality 4.0, enabling manufacturers to harness the power of virtual replicas and advanced analytics to move towards near-zero defect manufacturing. Manufacturers can acquire a better understanding of their products by virtualized representation of the actual product or asset and enable organizations to test future actions that could improve asset performance by merging AI techniques with digital twins.
There are typically 4 applications of digital twins in zero-defect manufacturing:
Wrapping Up
Finally, the integration of AI in manufacturing is making the concept of zero defect a reality. Companies that use AI technologies are realizing the full potential of their investments by seamlessly integrating virtual and physical assets. AI can monitor and analyze industrial processes in real time, identifying performance issues and opportunities to improve quality and throughput.
For those who are unclear about where to begin, our expertise is available to guide and assist in implementing AI-driven solutions, paving the way for a more efficient, high-quality, and defect-free manufacturing environment.