Editor’s Note: Whether you're a data-driven organization seeking the perfect solution or an industry enthusiast hungry for insights, join us on this enlightening journey as we decode the secrets of AWS, Azure, Snowflake, and GCP, empowering you to make data-driven decisions. Get ready to revolutionize your data management strategy and unlock limitless possibilities in the ever-evolving world of technology!
Introduction
Cloud technology can connect everything, and data is at the core of this connectivity. Technology serves as the mediator, facilitating the exchange of data and enabling seamless integration across different devices and systems. This interconnectedness revolutionizes the way businesses operate, creating new opportunities and challenges. Amidst this digital transformation, understanding the organization's position on the Data Maturity Curve become crucial.
Navigate Data Maturity Curve
Moving from a reactive to a predictive approach, the level of maturity in data and AI greatly impact the competitive advantage of large enterprises. The greater the level of maturity, the more successful they tend to be, thereby gaining an edge over their competitors. The journey towards data and AI maturity consists of various phases. Are you aware of your position on the Data Maturity Curve?
The data curve journey starts with cleaning the data from different data sources and then eventually leading to data exploration, and predictive analysis which will help in automated decision making which is the last stage.

If you see, step 1-4 is looking at the flashback to analyze what happened in the past. These phases rely on BI use cases from the data warehouse which holds historical data to produce valuable observations.
However, stage 5-7 relies on AI use-cases from data lake which helps companies understand and predict the future based on business constraints and how they can react in real-time. As companies progress in making automated decisions, they gain a competitive edge, leading to exponential business growth.
To combine BI and AI use cases together, companies strive to first ingest their data into a data lake, which caters to AI use cases. Subsequently, they proceed to ingest this data from the data lake into the data warehouse, specifically designed for BI use cases. This process is depicted in the diagram below, illustrating the sequential data flow.
Now that we understand the data maturity flow, the question comes back to where to store it. As you might be aware, data is generally stored in Data Warehouse and Data Lake. Each of them has its own challenges.
And keeping the data in two different platforms – Data Warehouse and Data Lake has its own challenges like duplication, synchronization of data, collaboration, security & governance, etc.

Both systems - Data Warehouse and Data Lake have advantages, but running parallel systems while going from reactive to predictive analysis introduces complexity that slows data operations. This complexity creates 3 major challenges:
1. Disjointed & duplicate data silos – 90-95% of the data in organisations is unstructured which lands in a data lake as it processes both structured & unstructured data whereas Data warehouse processes only structured data – creating duplicate, out-of-sync data.
2. Incompatible security & governance models – Both platforms offer different governance models that are not compatible with each other.
3. Different data on different platforms – Data warehouse relies on BI use cases whereas data lake relies on AI use cases which stands outperforming differently.
After seeing all the challenges of operating on two different platforms, what if companies can do all on one platform having one security & governance model?
Introducing Lakehouse: Unified platform
For all AI, ML, SQL, and Streaming use cases data lakehouse is a new, open system design that incorporates similar data structures and management features found in traditional data warehouses, directly on the cost-efficient storage used for data lakes.
By merging these capabilities into a single system, data teams can accelerate their operations, as they no longer need to access multiple systems to utilize data. It also ensures that teams have access to the most comprehensive and current data for their persona-based use cases of data science, machine learning, and business analytics.
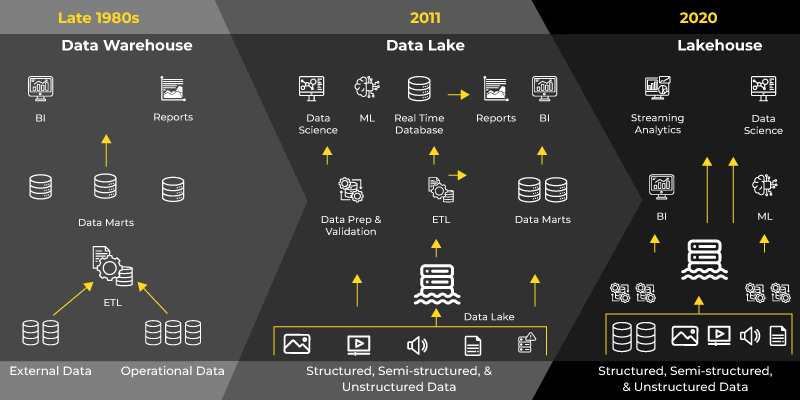
4 Major Players Face-off: Data Industry
Let's examine the key players in the cloud data storage industry. The major players include Amazon Web Services (AWS), Microsoft Azure, Google Cloud, and Snowflake. While IBM and Oracle also offer their own solutions, we will focus on the "big four" providers for now, as their offerings operate similarly.
Amazon Web Services emerged in 2006 as a spin-off of Amazon's extensive data center infrastructure. Google Cloud, its notable competitor, entered the scene in April 2008, followed by Microsoft Azure in October 2008. Snowflake, the newest entrant, was established in 2012. At first glance, AWS seems to have an advantage in terms of longevity.
However, this initial dominance did not last. Microsoft swiftly adapted to the competition from a non-IT rival. Snowflake, founded by three experts in data storage, quickly innovated and expanded its services. Instead of creating an entire cloud provider platform, Snowflake focused on delivering an intuitive experience that could be deployed to any major cloud, abstracting the technical complexities that often hinder integration and scalability. While Google Cloud took some time to develop and offer additional services but as they expanded their own internal products, they expanded cloud services as well.
When it comes to market share – Amazon has captured 33% followed by Azure with 21%, and Google holds 8%, while the remaining market share is divided among other competitors. Amazon's substantial lead in the market is not a surprise. Let's delve into a detailed comparison of their features and assess how they differ from one another.
Game of Comparison: AWS vs Snowflake vs Google Cloud vs Azure
Let’s get started with the comparison game!
Now that we have gained an understanding of the current market positions of these four major players in the cloud industry, let’s also explore the difference in their multitude of offerings.
1. Microsoft Azure
PRICING | FEATURES | DOWNSIDE |
---|---|---|
Azure divides its services into compute and storage charges. When the service is paused, the customer has to incur only storage cost. It doesn’t charge upfront costs and termination fees. |
PRICING | FEATURES | DOWNSIDE |
---|---|---|
A tiered pricing strategy that is customized to suit individual needs and preferences as it offers pricing plans that cater to both pre-purchase arrangements and on-demand usage. The usage of compute and storage are separated, and compute is billed separately on a per-second basis. |
3. Amazon Web Services (AWS)
PRICING | FEATURES | DOWNSIDE |
---|---|---|
AWS offers an affordable starting point with their free tier, allowing users to build proof of concepts without incurring any costs. However, the true costs of AWS products become apparent when they are utilized in production environments. |
4. Google Cloud Platform (GCP)
PRICING | FEATURES | DOWNSIDE |
---|---|---|
Pay-as-you-go model, which means companies are charged for the actual resources consumed. Billed based on the usage duration and the quantity of resources utilized. |
Ideal Lakehouse Platform: Which one is right for you?
When it comes to selecting the ideal Lakehouse platform, enterprises must carefully evaluate the distinguishing features of each option. This comprehensive analysis will enable them to make an informed decision that aligns with their specific needs and requirement.
Features | AWS | Azure | Snowflake | GCP |
---|---|---|---|---|
Architecture | AWS Glue is a fully managed extract, transform, and load (ETL) service. Automated data discovery, cataloging, and schema inference capabilities. | Azure Synapse combines data warehousing, big data, and data integration capabilities. Allows for data ingestion, preparation, exploration, and serving analytical queries. | It combines the traditional shared disk with the shared-nothing database architectures. Snowflake consists of database storage, query processing, and cloud services. | Google BigQuery provides a serverless data warehouse for running fast and scalable analytics on structured and semi-structured data. |
Integration | Services like AWS Data Pipeline and AWS AppSync, which facilitate data integration and enable real-time data streaming and synchronization. | Azure Data Factory helps orchestrate and automate data workflows across various sources and destinations. It supports data ingestion, transformation, and loading into a Lakehouse architecture. | Provides native connectors and integrations and supports popular data integration tools like Apache Kafka, Apache NiFi, and more. It also offers integrations with data engineering platforms like Fivetran, Matillion, and Talend etc. | Cloud Data Fusion provides a visual interface for building data integration pipelines.BigQuery for data warehousing and Cloud Pub/Sub for real-time messaging and streaming data integration. |
Security | Both the user and AWS are responsible for securing data. | Azure uses access management, information security, threat protection, network security, and data protection for data security. It also has over 90 compliance certificates. | Snowflake complies with many data protection standards and has implemented controlled access management and data security by encrypting all data and files. | Google Cloud Identity and Access Management (IAM) for fine-grained access control, Cloud Security Command Center for centralized security monitoring, and Cloud Key Management Service (KMS) for encryption key management. |
Data Backup & Recovery | Amazon S3 (Simple Storage Service) for data storage and backup, and Amazon Glacier for long-term archival storage. AWS Backup provides a centralized backup management solution | Azure Backup automates backups for virtual machines, databases, and files, ensuring long-term retention and easy data recovery. Azure Site Recovery replicates applications and virtual machines, ensuring continuous operations and quick failover. | Snowflake does not provide traditional backup and recovery mechanisms, as it relies on its built-in data replication and storage architecture. | Google Cloud Storage for data storage and backup, and Cloud Snapshot Manager for managing and scheduling backups. |
Security | Both the user and AWS are responsible for securing data. | Azure uses access management, information security, threat protection, network security, and data protection for data security. It also has over 90 compliance certificates. | Snowflake complies with many data protection standards and has implemented controlled access management and data security by encrypting all data and files. | Google Cloud Identity and Access Management (IAM) for fine-grained access control, Cloud Security Command Center for centralized security monitoring, and Cloud Key Management Service (KMS) for encryption key management. |
Enterprise Suitability | AWS is known for its extensive service portfolio and mature cloud offerings. Suitable for enterprises with diverse workloads and applications. | Cost-effective data warehouse solution that doesn't compromise on performance, making it a great choice for enterprises heavily relied on Microsoft technologies. | Snowflake is a perfect fit for enterprises in need of a scalable and fully managed solution that comes with built-in performance optimization. | Appealing to organizations focusing on data-driven insights and AI applications as it offers integration with popular Google technologies, such as BigQuery and TensorFlow |
Ultimately, the decision boils down to finding the right balance between the must-have features, scalability potential, and personal preferences. By carefully weighing these factors, companies can make an informed choice that sets their enterprise on the path toward leveraging the full potential of a Lakehouse platform.
Snowflake vs AWS vs Azure (Conclusion)
Congratulations on investing the time to explore this topic thoroughly. We trust that you are now well-informed about the distinctions among AWS vs Snowflake vs Azure vs Google Cloud. This empowers you to make informed decisions that will drive your enterprise's success and enable you to harness the power of data effectively.
But if you’re still confused about the ideal Data Lakehouse for your enterprise. Get Expert Help from Polestar Solutions to determine which Data Lakehouse is the best fit for your organization.
Get in touch with our team for a free consultation regarding your data warehousing requirements.