Editors Note: Managing Data pipelines (or quality) can be a headache. In this blog, we will talk about Data Observability. Imagine X-ray vision for your data pipelines, detecting anomalies, ensuring quality, and preventing disasters before they hit. Learn the 5 pillars of data observability and get the trust that you want from your data. Learn how AI can power your insights, and help you in real-time monitoring of your data. Take control of your data health today!
If you have been asking yourself the following questions constantly then you might want to take a look at your data practices and this blog will help you know more about how you can fix your problem.
→ Is the data up to date?
→ Isn't this value suspiciously high?
→ Why are there so many nulls?
→ Why do we have duplicate IDs?
What reports will I break with a schema update?
Imagine this: It's Tuesday morning, and you're the data champion in your IT team. Suddenly, your phone explodes with frantic calls – reports are going haywire, dashboards are showing alarming trends, and key business decisions are on hold. The culprit? Bad data.
Here's where Data Observability comes in as your superhero sidekick. Instead of scrambling blindly, you'd have a complete X-ray of your data ecosystem. You'd know:
With this deep understanding, you could:
So, No more Tuesday morning meltdowns. Instead, you'd be the data guru, calmly navigating the complexities of your ecosystem, ensuring every decision is powered by reliable, trustworthy information. Now, that's a superpower worth having!
The Impact of Data Observability on Enhancing Data
Data observability is the practice of monitoring and understanding the quality, reliability, and performance of your data. It involves tracking and analyzing data in real-time to ensure that it is accurate, complete, and consistent. By implementing data observability, organizations can gain valuable insights into their data and make informed decisions.
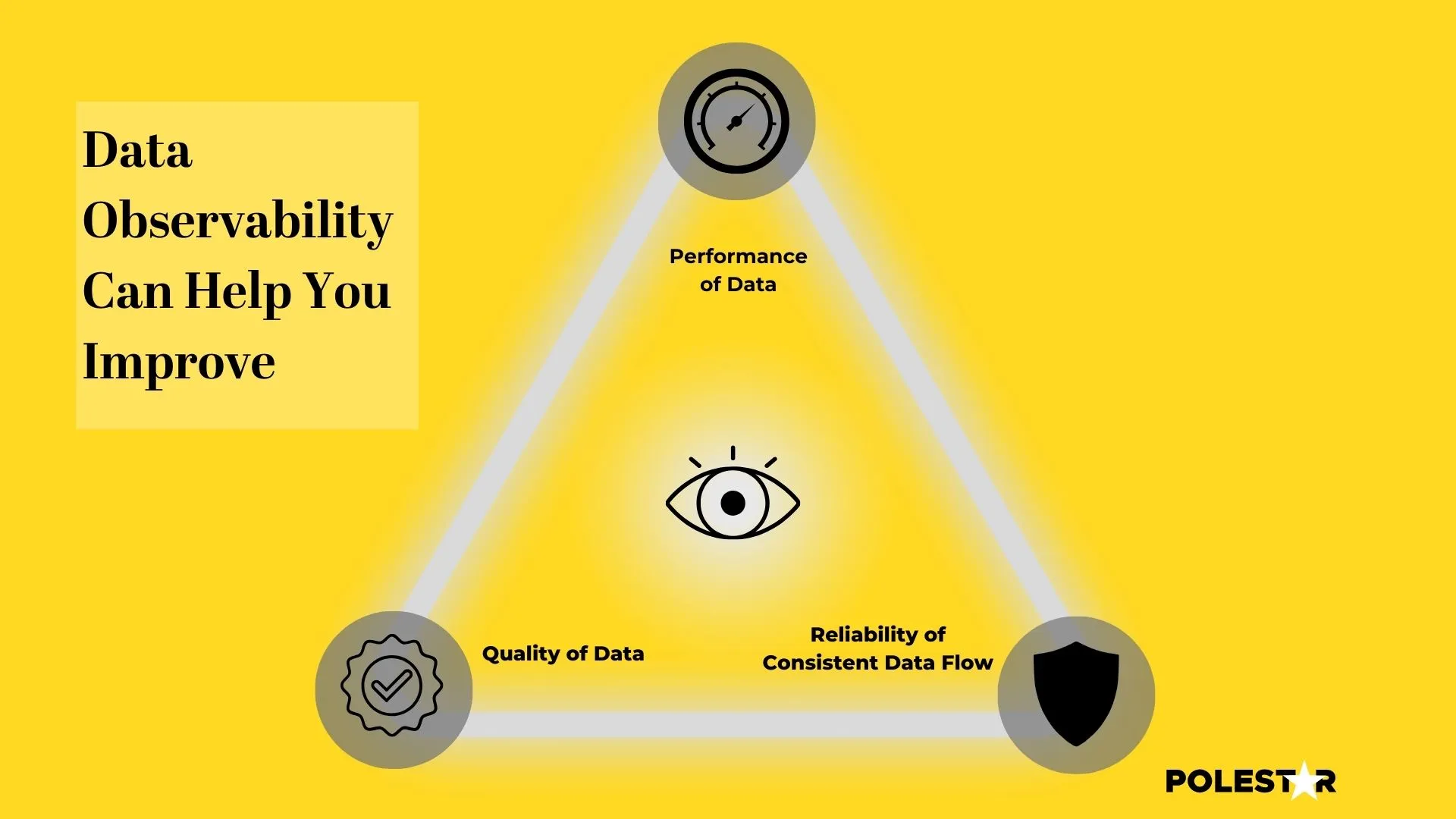
One of the key aspects of data observability is data quality. It is essential to ensure that the data being collected and processed is of high quality and meets the required standards. Data observability helps in identifying and resolving issues related to data quality, such as missing or incorrect data, duplicate entries, and data inconsistencies.
Another important aspect of data observability is data reliability. Organizations need to ensure that the data they rely on is trustworthy and can be used for critical decision-making. Data observability helps in monitoring data sources, detecting anomalies, and ensuring the reliability of data pipelines.
Data observability also plays a crucial role in ensuring the performance of data systems. It helps in monitoring the performance of data pipelines, identifying bottlenecks, and optimizing data processing to enhance overall system performance.
It is important to understand that data performance management and data observability are intertwined partners. They are not to be confused with each other, while performance management tracks the pulse of your data ecosystem, observability acts as the X-ray, revealing its internal health and potential vulnerabilities.
According to Gartner, By 2026, 30% of enterprises implementing distributed data architectures will have adopted data observability techniques to improve visibility over the state of the data landscape, up from less than 5% in 2023.
To get started, focus on these 5 Pillars
The five pillars of freshness, quality, volume, schema, and lineage, empower you to optimize data pipelines, prevent issues before they surface, and unlock the true potential of your data for informed decision-making.
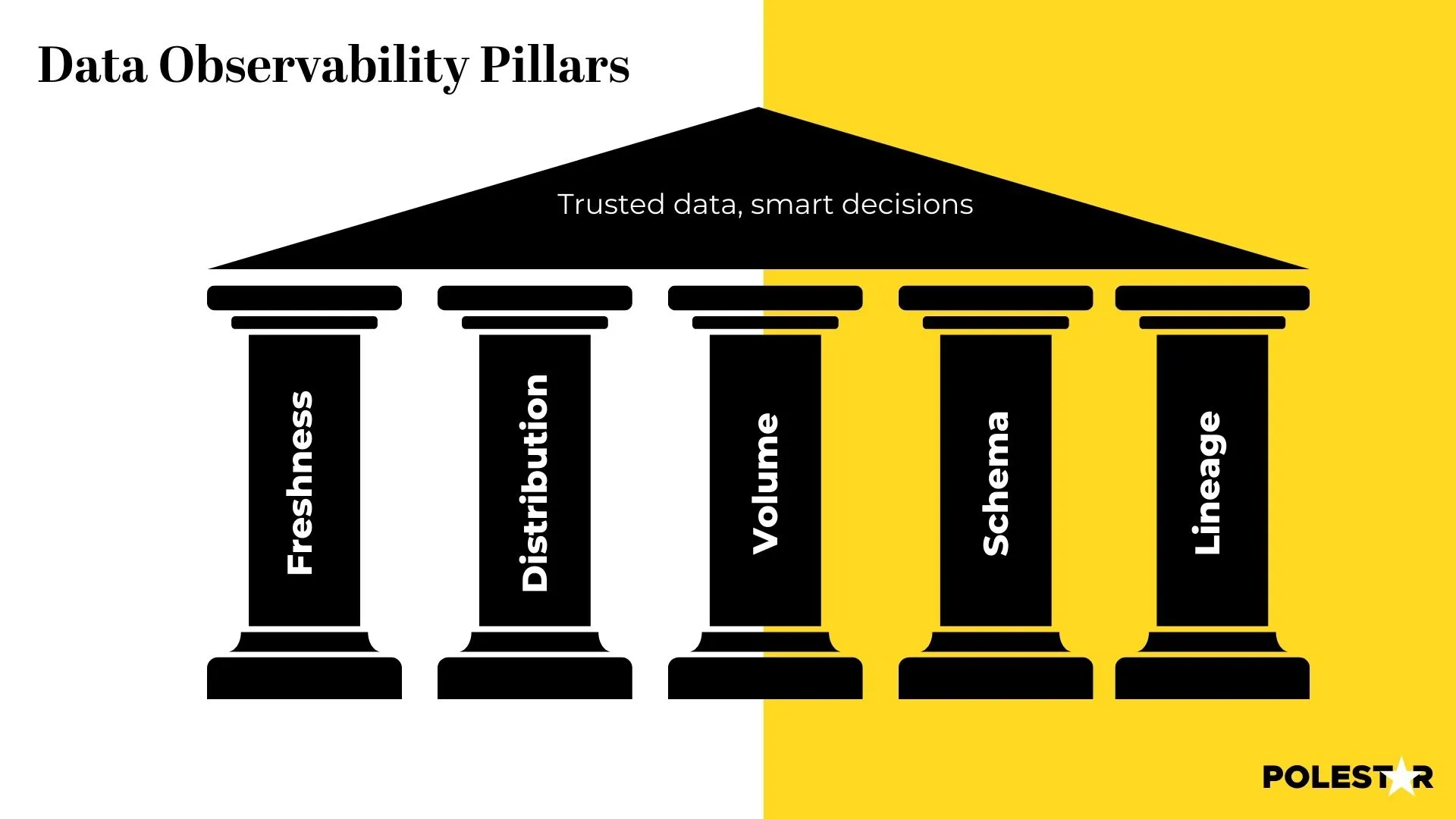
Automated Monitoring for Freshness: Ensuring data is up-to-date and less prone to decay is a priority, it allows for timely identification and resolution of potential issues.
Distribution KPIs: Distribution is monitored for adherence to trusted ranges, maintaining data integrity and reliability.
Volume and completeness: Metrics ascertain the availability of essential data.
Schema: Defines the structure of the data, including the types of data, relationships, and constraints, makes sure data is structured, organized, and interpretable.
Lineage: Pinpointing the source of problems, aiding swift resolutions.
Data observability is frequently viewed predominantly through the perspective of data quality, with some considering the terms interchangeable. Although there are similarities between the two concepts, distinctions exist. Data quality primarily focuses on the data itself, whereas data observability extends its concerns to encompass the system and environment responsible for delivering the data.
Steppingstones of Observability
→ Monitor and detect: Data observability gathers and analyzes signals from diverse channels, offering a comprehensive perspective on the performance of data pipelines. It assesses data quality, identifies issues, and ensures seamless operation.
→ Alert and troubleshoot: It categorizes issues by urgency and severity, sending timely alerts for quick response. Root cause analysis is performed, enabling effective troubleshooting and resolution.
→ Recommendations: Tailored recommendations are provided based on the analysis—ranging from informative insights for legacy systems to urgent solutions for critical issues. This personalized approach distinguishes vendors.
→ Resolve and Prevent: By implementing recommendations, users can address issues, optimize data pipelines, and avert system downtime. This proactive approach ensures continuous data integrity and system stability.
Here’s what the ultimate state would look like
To achieve effective data observability, organizations should aim to get to this stage:
End-to-End Visibility: Ensure observability across data streams, lakes, and warehouses. Detect and address data issues early by understanding the entire data flow.
Flexible Data Format Support: Embrace a variety of data formats, including structured and semi-structured (e.g., JSON blobs). Comprehensive observability requires monitoring diverse data types.
Granular Validation Techniques: Move beyond one-dimensional statistics to validate individual data points and consider dependencies between fields. Univariate and multivariate validation ensures data accuracy at all levels.
Configurable Validators: Strike a balance between automated suggestions and manual configuration. Ensure scalability by allowing customization without complex coding requirements.
Multi-Cadence Validation: Support validation at different time horizons, including real-time. Adapt to diverse data update frequencies for timely insights.
User-Centric Approach: Cater to both technical and non-technical users. Provide multiple modes of control, democratizing data quality and fostering collaboration across departments.
Benefits of Implementing Data Observability
Implementing data observability offers numerous benefits for organizations. Some of the key benefits include:
1. Improved Data Quality: Data observability helps in monitoring and ensuring the quality of data, leading to improved accuracy and reliability of data.
2. Enhanced Data Reliability: By monitoring data sources and pipelines, organizations can ensure the reliability of data, enabling them to make critical decisions with confidence.
3. Faster Issue Detection and Resolution: Data observability enables real-time monitoring of data, allowing organizations to quickly detect and resolve issues related to data quality, reliability, and performance.
4. Increased Data Visibility: Data observability provides organizations with visibility into their data pipelines, allowing them to identify bottlenecks, optimize data processing, and improve overall system performance.
Overall, implementing data observability can help organizations unlock the full potential of their data, improve operational efficiency, and gain a competitive edge in the market.
Future Trends in Data Observability
The field of data observability is constantly evolving, and several future trends are expected to shape its development. Some of the key future trends in data observability include:
1. AI-powered Data Observability: The use of artificial intelligence and machine learning techniques to enhance data observability and automate data monitoring and analysis.
2. Real-time Data Observability: The shift towards real-time data observability, enables organizations to monitor and analyze data in real-time, leading to faster issue detection and resolution.
3. Data Observability for Cloud and Hybrid Environments: The increased adoption of cloud and hybrid environments necessitates the development of data observability practices specifically designed for these environments.
4. Integration with Data Governance: The integration of data observability practices with data governance frameworks to ensure data compliance, privacy, and security.
5. Predictive Data Observability: The use of predictive analytics to anticipate and prevent data issues before they occur, improving data reliability and performance.
By embracing these future trends, organizations can stay ahead of the curve and leverage data observability to drive innovation and business success.We can assist
Leveraging our deep analytics expertise, you can seamlessly implement data observability measures. Our data discovery workshops unveil data flows and potential bottlenecks, facilitating strategic observability implementation.
We guide the selection and integration of optimal observability tools, tailoring solutions to meet specific data pipelines and quality requirements. With Polestar's comprehensive support, enterprises gain a clear view of their data health, paving the way for improved efficiency and data-driven decisions.
We help you implement “Automated observability” for cloud-native and hybrid environments so that your cloud complexity gets simplified.